Resources
Ecomics
Ecomics is a consistent, quality-controlled multi-omics compendium for Escherichia coli with cohesive meta-data information.
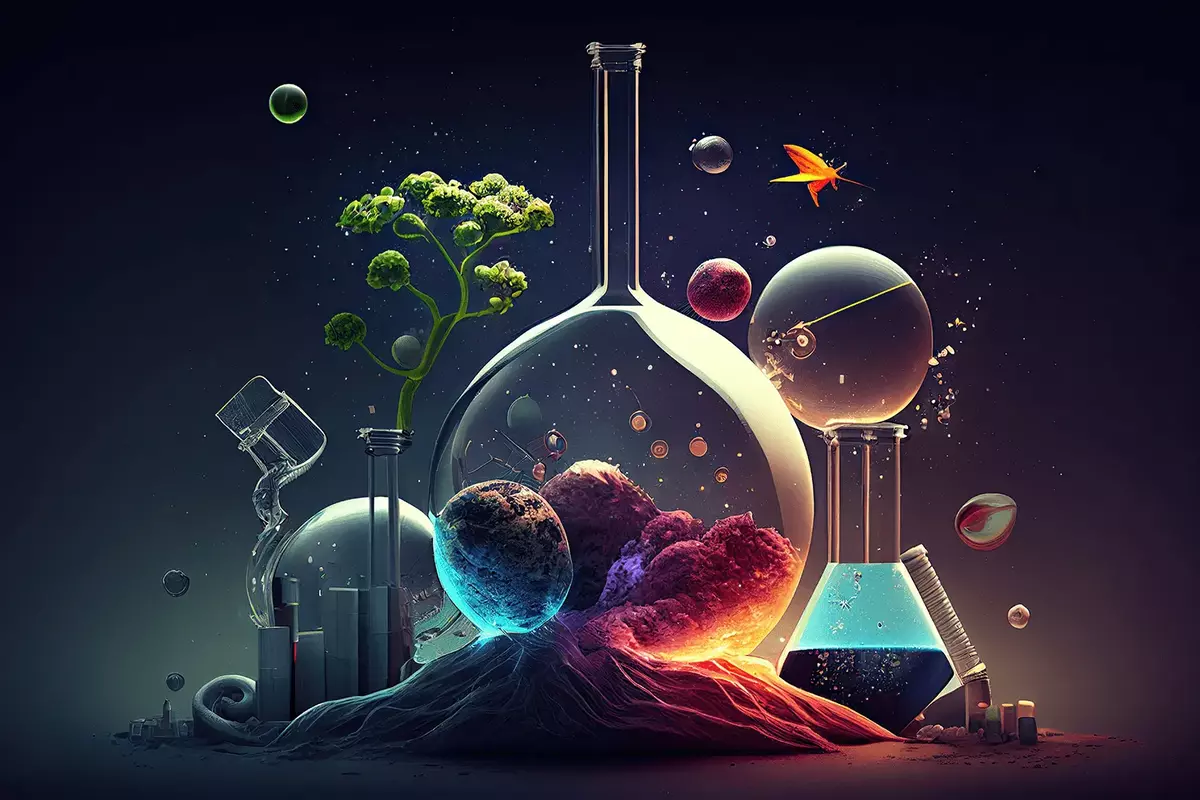
We then use this resource to train a multi-scale model that integrates four omics layers to predict genome-wide concentrations and growth dynamics. The genetic and environmental ontology reconstructed from the omics data is substantially different and complementary to the genetic and chemical ontologies. The integration of different layers confers an incremental increase in the prediction performance, as does the information about the known gene regulatory and protein-protein interactions. The predictive performance of the model ranges from 0.54 to 0.87 for the various omics layers, which far exceeds various baselines. This work provides an integrative framework of omics-driven predictive modelling that is broadly applicable to guide biological discovery.
Reference:
Kim, M., Rai, N., Zorraquino, V., & Tagkopoulos, I. (2016). Multi-omics integration accurately predicts cellular state in unexplored conditions for Escherichia coli. Nature communications, 7(1), 1-12.
MutationDB
MutationDB is a coherent compendium of more than 15,000 mutation events for the bacterium Escherichia coli under 178 distinct environmental settings.
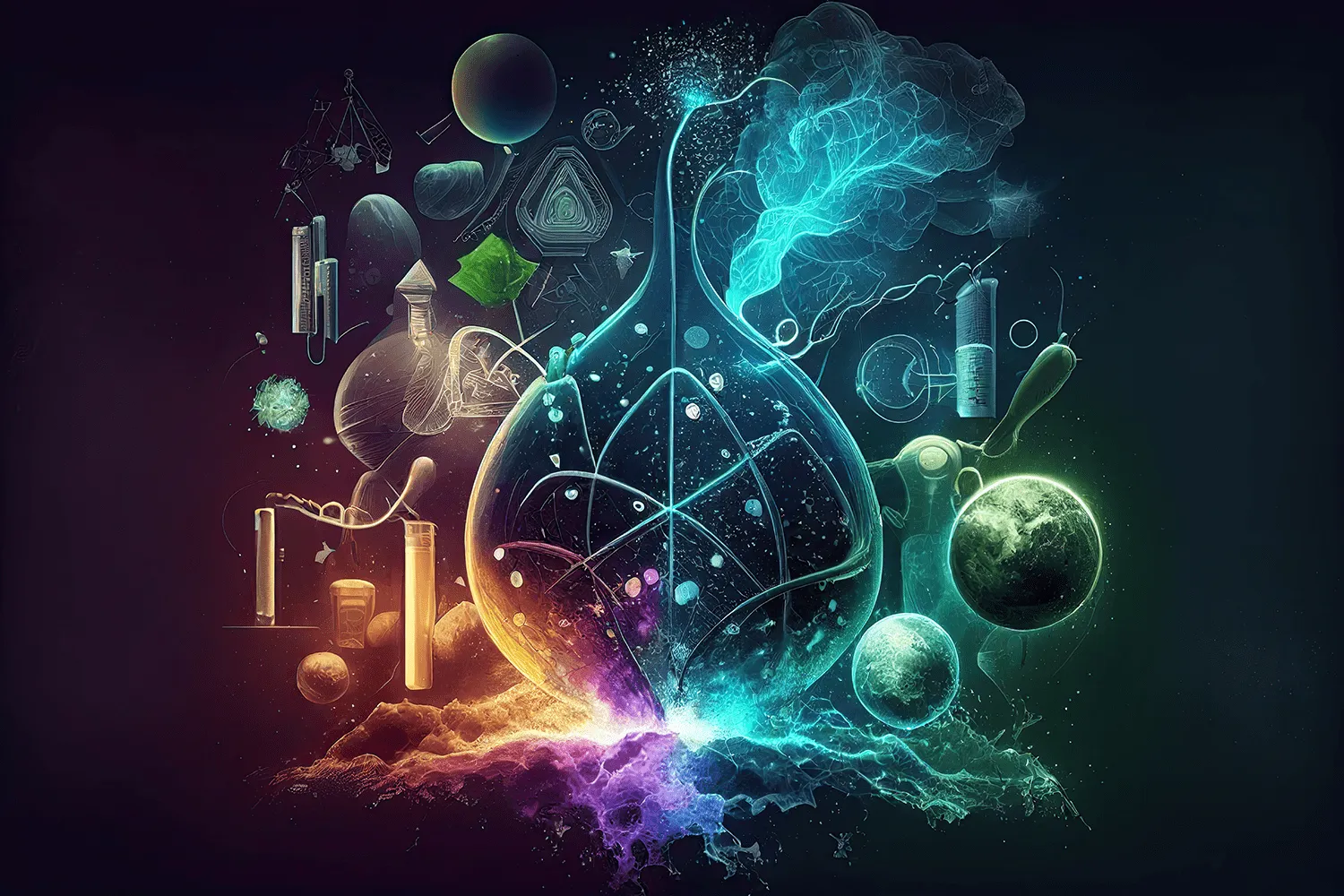
Compendium analysis provides a comprehensive view of the explored environments, mutation hotspots and mutation co-occurrence. While the mutations shared across all replicates decrease with the number of replicates, our results argue that the pairwise overlapping ratio remains the same, regardless of the number of replicates. An ensemble of predictors trained on the mutation compendium and tested in forward validation over 35 evolution replicates achieves a 49.2 ± 5.8% (mean ± std) precision and 34.5 ± 5.7% recall in predicting mutation targets.
Reference:
Wang, X., Zorraquino, V., Kim, M., Tsoukalas, A., & Tagkopoulos, I. (2018). Predicting the evolution of Escherichia coli by a data-driven approach. Nature communications, 9(1), 1-12.
PAMDB
PAMDB integrates data from 135 publications that contain 118 circuits and 165 genetic parts of the bacterium Escherichia coli.
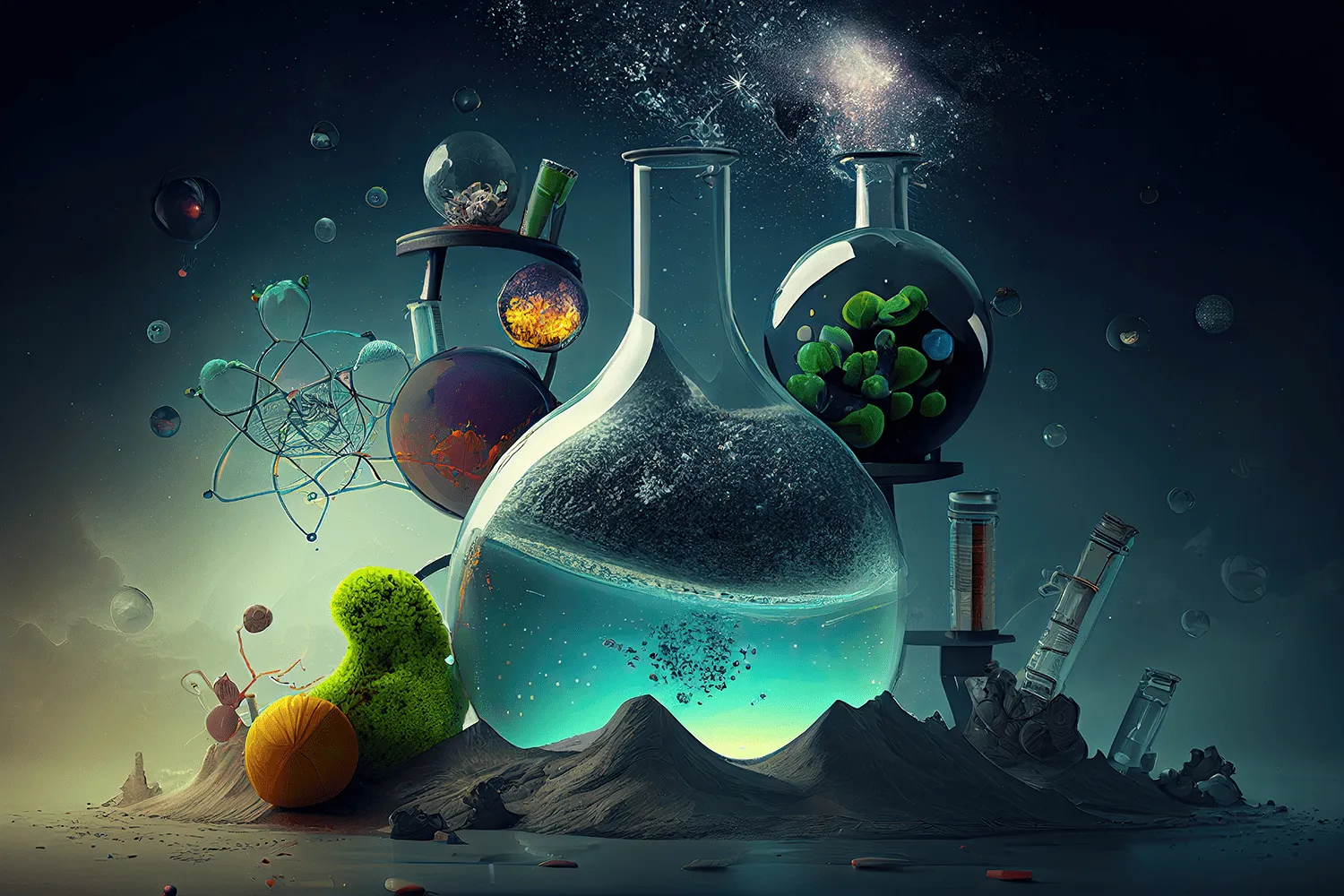
We used a succinct, universal model formulation to describe the part behavior in each circuit. We introduce a constrained consensus inference method that was used to infer the value of the model parameters and evaluated its performance through cross-validation in a benchmark of 23 circuits. This work provides a resource and a methodology that can be used as a point of reference for synthetic circuit modeling.
Reference:
Huynh, L., & Tagkopoulos, I. (2016). A parts database with consensus parameter estimation for synthetic circuit design. ACS Synthetic Biology, 5(12), 1412-1420.