New article in Nature Communications
A new publication from Jason Youn, Dr. Navneet Rai, and Dr. Ilias Tagkopoulos, about Knowledge integration and decision support for accelerated discovery of antibiotic resistance genes.
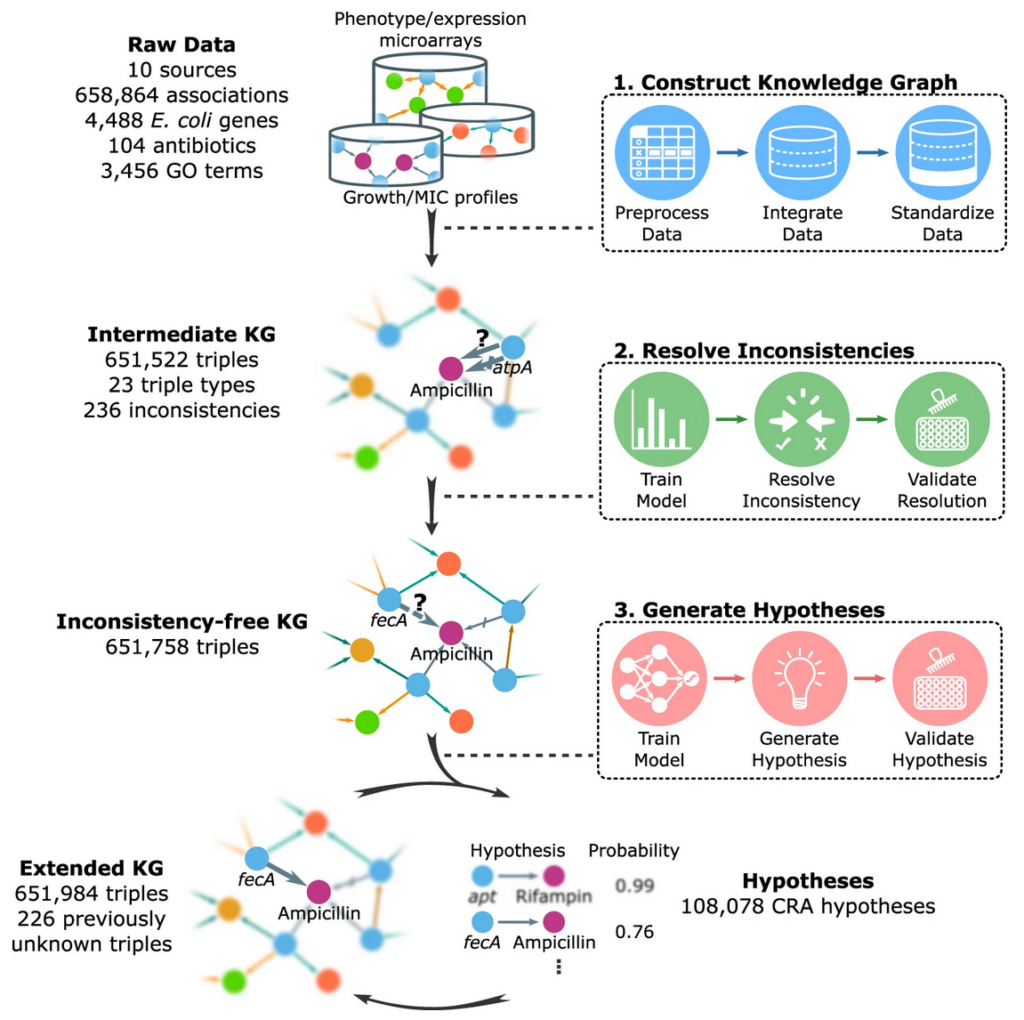
Abstract: We present a machine learning framework to automate knowledge discovery through knowledge graph construction, inconsistency resolution, and iterative link prediction. By incorporating knowledge from 10 publicly available sources, we construct an Escherichia coli antibiotic resistance knowledge graph with 651,758 triples from 23 triple types after resolving 236 sets of inconsistencies. Iteratively applying link prediction to this graph and wet-lab validation of the generated hypotheses reveal 15 antibiotic resistant E. coli genes, with 6 of them never associated with antibiotic resistance for any microbe. Iterative link prediction leads to a performance improvement and more findings. The probability of positive findings highly correlates with experimentally validated findings (R2 = 0.94). We also identify 5 homologs in Salmonella enterica that are all validated to confer resistance to antibiotics. This work demonstrates how evidence-driven decisions are a step toward automating knowledge discovery with high confidence and accelerated pace, thereby substituting traditional time-consuming and expensive methods.
Reference: Youn, Jason, Navneet Rai, and Ilias Tagkopoulos. “Knowledge integration and decision support for accelerated discovery of antibiotic resistance genes.” Nature Communications (2022). doi: 10.1038/s41467-022-29993-z (link), (GitHub)