New article in Scientific Reports
A new publication from collaborators and Dr. Ilias Tagkopoulos, about An Early Prediction Model for Canine Chronic Kidney Disease Based on Routine Clinical Laboratory Tests.
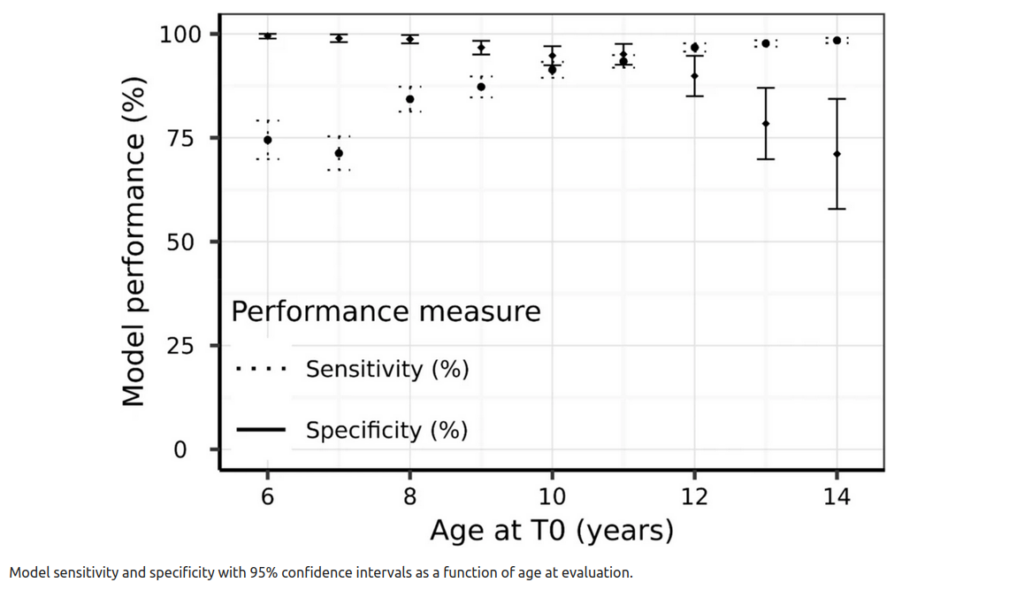
Abstract: The aim of this study was to derive a model to predict the risk of dogs developing chronic kidney disease (CKD) using data from electronic health records (EHR) collected during routine veterinary practice. Data from 57,402 dogs were included in the study. Two thirds of the EHRs were used to build the model, which included feature selection and identification of the optimal neural network type and architecture. The remaining unseen EHRs were used to evaluate model performance. The final model was a recurrent neural network with 6 features (creatinine, blood urea nitrogen, urine specific gravity, urine protein, weight, age). Identifying CKD at the time of diagnosis, the model displayed a sensitivity of 91.4% and a specificity of 97.2%. When predicting future risk of CKD, model sensitivity was 68.8% at 1 year, and 44.8% 2 years before diagnosis. Positive predictive value (PPV) varied between 15 and 23% and was influenced by the age of the patient, while the negative predictive value remained above 99% under all tested conditions. While the modest PPV limits its use as a stand-alone diagnostic screening tool, high specificity and NPV make the model particularly effective at identifying patients that will not go on to develop CKD.
Reference: Kokkinos, Yiannis, JoAnn Morrison, Richard Bradley, Theodoros Panagiotakos, Jennifer Ogeer, Dennis Chew, Ciaran O’Flynn, Geert De Meyer, Phillip Watson, and Ilias Tagkopoulos. “An early prediction model for canine chronic kidney disease based on routine clinical laboratory tests.” Scientific Reports (2022). doi: s41598-022-18793-6 (link)