New article in Journal of the American Medical Informatics Association
A new publication from Dr. Ameen Eetemadi and Dr. Ilias Tagkopoulos, about Algorithmic Lifestyle Optimization.
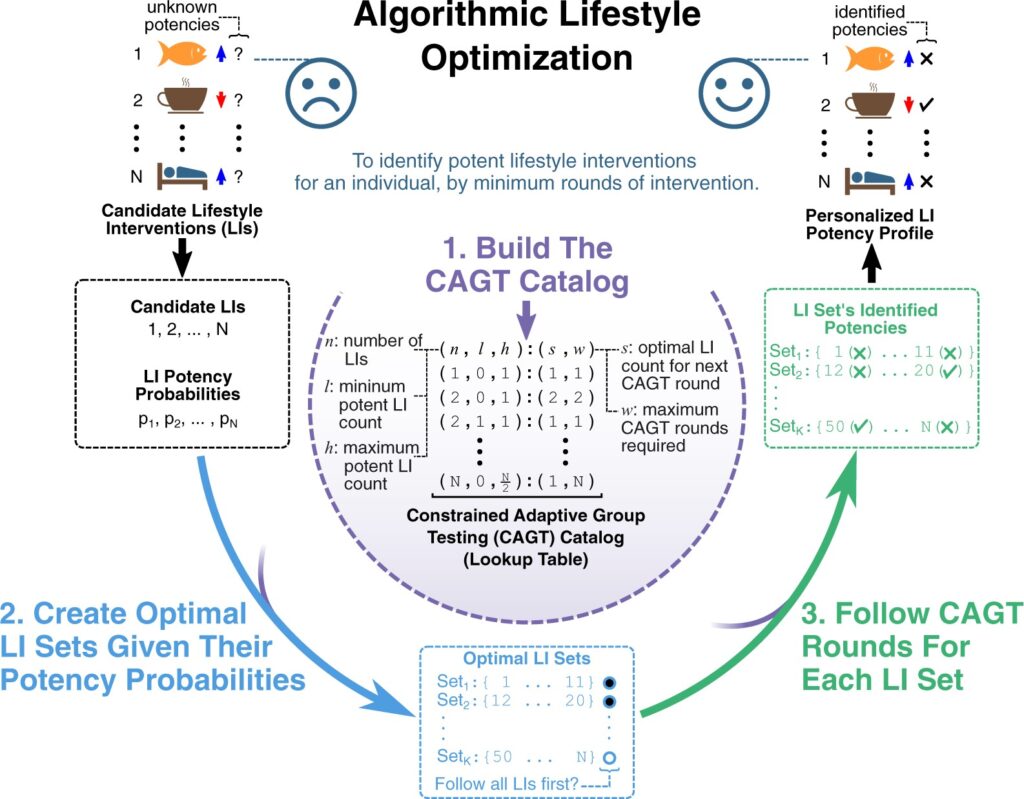
Abstract: A hallmark of personalized medicine and nutrition is to identify effective treatment plans at the individual level. Lifestyle interventions (LIs), from diet to exercise, can have a significant effect over time, especially in the case of food intolerances and allergies. The large set of candidate interventions, make it difficult to evaluate which intervention plan would be more favorable for any given individual. In this study, we aimed to develop a method for rapid identification of favorable LIs for a given individual. We have developed a method, algorithmic lifestyle optimization (ALO), for rapid identification of effective LIs. At its core, a group testing algorithm identifies the effectiveness of each intervention efficiently, within the context of its pertinent group. Evaluations on synthetic and real data show that ALO is robust to noise, data size, and data heterogeneity. Compared to the standard of practice techniques, such as the standard elimination diet (SED), it identifies the effective LIs 58.9%–68.4% faster when used to discover an individual’s food intolerances and allergies to 19–56 foods.
Reference: Eetemadi, Ameen, Ilias Tagkopoulos. “Algorithmic lifestyle optimization.” Journal of the American Medical Informatics Association (2022). doi: 10.1093/jamia/ocac186 (link), (pdf), (GitHub coming soon)