New article in Journal of Agricultural and Food Chemistry
A new publication from Cheng-En Tan, Prof. Ilias Tagkopoulos, and collaborators about Volatile organic compound-based predictive modeling of smoke taint in wine.
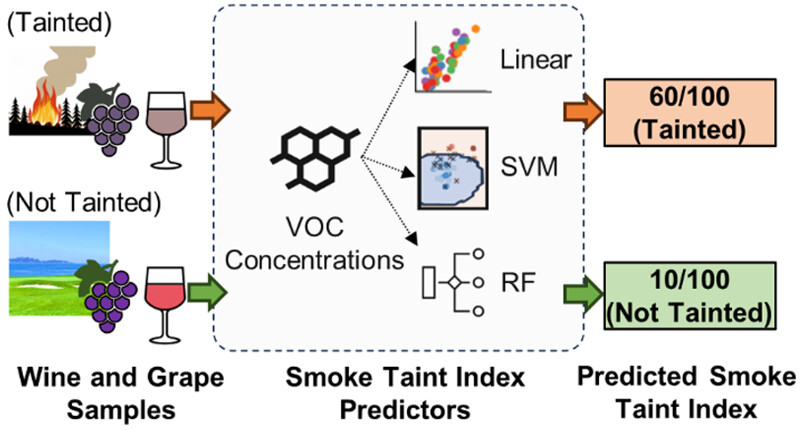
Abstract: Smoke taint in wine has become a critical issue in the wine industry due to its significant negative impact on wine quality. Data-driven approaches including univariate analysis and predictive modeling are applied to a data set containing concentrations of 20 VOCs in 48 grape samples and 56 corresponding wine samples with a taster-evaluated smoke taint index. The resulting models for predicting the smoke taint index of wines are highly predictive when using as inputs VOC concentrations after log conversion in both grapes and wines (Pearson Correlation Coefficient PCC = 0.82; R2 = 0.68) and less so when only grape VOCs are used (Pearson Correlation Coefficient PCC = 0.76; R2 = 0.56), and the classification models also show the capacity for detecting smoke-tainted wines using both wine and grape VOC concentrations (Recall = 0.76; Precision = 0.92; F1 = 0.82) or using only grape VOC concentrations (Recall = 0.74; Precision = 0.92; F1 = 0.80). The performance of the predictive model shows the possibility of predicting the smoke taint index of the wine and grape samples before fermentation. The corresponding code of data analysis and predictive modeling of smoke taint in wine is available in the Github repository (https://github.com/IBPA/smoke_taint_prediction).
Reference: Tan, Cheng-En, Bishnu Prasad Neupane, Yan Wen, Lik Xian Lim, Cristina Medina Plaza, Anita Oberholster, and Ilias Tagkopoulos. “Volatile Organic Compound-Based Predictive Modeling of Smoke Taint in Wine.” Journal of Agricultural and Food Chemistry (2024). doi: 10.1021/acs.jafc.3c07019 (link) (GitHub)